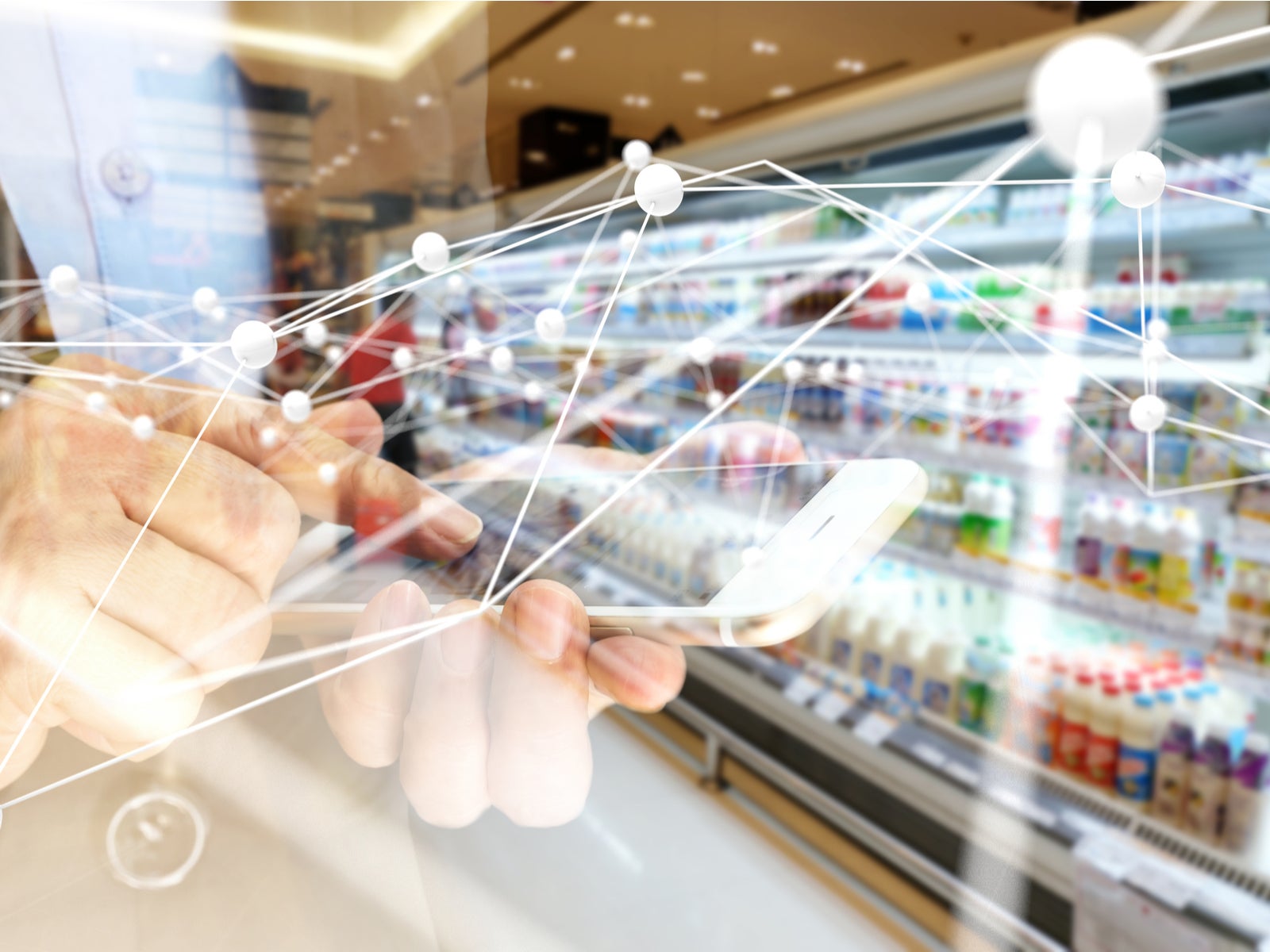
Predictive analytics is a hot topic in the fast-moving consumer goods industry (FMCG) and something that manufacturers and investors look for to determine which products will be successful.
There are two key aspects to consider when it comes to predictive analysis: the quality of data and machine learning segmentation. Establishing an enriched data hub from a comprehensive pipeline of data sources that also offers highly relevant insight is the first step to attaining effective market insight; while using an effective combination of analysis methods to visualise and understand this data is key to making investment and development decisions.
Data analytics company Signal Analytics uses integrated artificial intelligence (AI), natural language processing (NLP) and machine learning (ML) as well as human analysis to draw from a high-quality pool of collated data. According to the company’s director of data strategy, Omer Kehat: “When we collect data, we make sure that it is relevant to the vertical and business questions we are aiming to address. ML allows us to run data through various filters to keep only the data that is needed for the analytic task at hand.”
Precise predictive analytics
This means that overlaps in terms or product descriptions don’t disrupt data outcomes. For example, data for skincare companies collected from e-commerce sites will need to include cosmetics face masks, but exclude face coverings. The data collected by Signal Analytics is so enriched that it is very easy for a customer to tailor the platform to suit their needs.
As Kehat explains: “We are a data platform, that incorporates all the elements of a data fabric. This means that we manage the entire flow from ingesting, classifying, and managing the data and are responsible for data governance from end-to-end. Our platform collects the data from various sources that we have researched and found to be relevant and comprehensive, for providing a holistic view of a specific category or ecosystem, such as food or beverage, or other consumer goods like beauty, personal care and so on.”
Signal Analytics uses CoreNLP, developed by Stanford University, on top of which it layered its own patented NLP techniques that structures the data in a more meaningful way, such as capturing correlations between negative and positive reviews.
How well do you really know your competitors?
Access the most comprehensive Company Profiles on the market, powered by GlobalData. Save hours of research. Gain competitive edge.
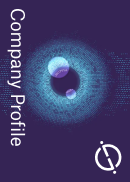
Thank you!
Your download email will arrive shortly
Not ready to buy yet? Download a free sample
We are confident about the unique quality of our Company Profiles. However, we want you to make the most beneficial decision for your business, so we offer a free sample that you can download by submitting the below form
By GlobalData“Once the proper data is ingested, the next step is putting it through our proprietary NLP algorithms, to extract context and sentiment with very high accuracy,” Kehat says. “Any ML algorithm is really going to be bound by how much segmentation it can give you. A typical ML algorithm might take a data set and give you some recommendations to cluster all of the data points into five different groups, based on differentiating factors, but ultimately a user is limited by what that algorithm can find out by itself.”
“We don’t want to be limited by an algorithm. We want to make sure that the algorithm works based on the way that our customer’s and the market views the data, down to the level of very specific attributes that they care about and want to track. We invest a lot of effort into building our own proprietary taxonomy for each category we focus on, including different dimensions and how deep those dimensions can run. The taxonomy reflects the attributes, benefits and features associated with a given product, and we want to make sure that when the insights are presented to the customer, they are specific enough to be relevant and actionable to them.”
Integrating it all
Signal Analytics combines this ML, bottom-up approach with a top-down approach, which involves human market research covering different industries so they can understand difference terms, lingos and trends. This combination of qualitative expert data and quantitative big data creates richer market insight and much more accurate predictive analytic results.
By combining so many different data sources, the accuracy of our predictive analytics jumps significantly,” Kehat adds. “Most platforms take historical sales models and predict future outcomes but we are able to connect those sales models to other data sources like social listening, product reviews, patents, blogs, forums and more, and get a much more holistic perspective of what is likely to happen in the future. This arms our customers with very specific market intelligence they can take to make decisions.”